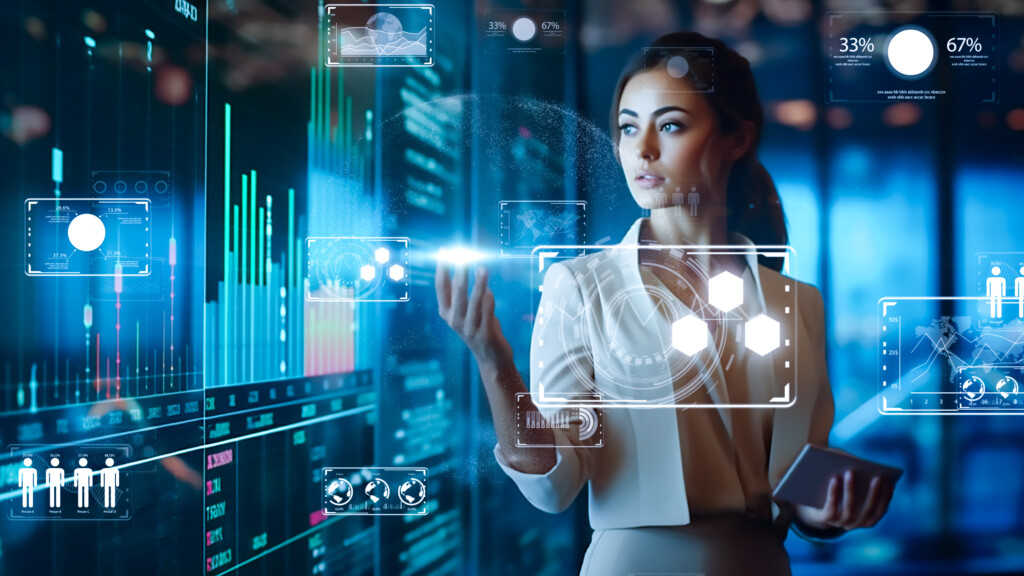
Competitiveness is increasing in many sectors. In some environments, overcapacities are contributing to this, in others suppliers from low-wage countries. But many companies are also struggling with locations in low-wage countries, because they have to position themselves on changing global markets.
Of course, there is classic potential for improvement in all companies, which can be used to reduce costs and bring attractive products to market. In addition, new technologies create further opportunities to increase efficiency and innovate business models.
Such opportunities can often only be tapped with the digitization of business processes and the integration and evaluation of process data. You can find out what specific possibilities there are in this article.
The following aspects should be considered:
- A digitization strategy with reference to the business
- The benefit-oriented digitization of business processes
- The collection and evaluation of relevant process data
- The opportunities offered by IIoT and cloud computing
- Data management requirements, including data integration
- The possibilities of evaluating Big Data with business analytics
- Special infrastructure tasks
- Robotics and plant automation
- Optimizing quality and increasing efficiency with computer vision applications
- Cost-effective process modeling and simulation with Digital Twins
These aspects are discussed in more detail below.
Digitalization in Operations
“Digitization” has become a buzzword. But few who use the term know what it actually entails and what is relevant.
To unlock the benefits of digitization, it must be approached strategically and done systematically.
Digitalization Strategy
The important first step toward digitization is therefore to develop a coherent digitization strategy that is suitable for supporting the business, reducing costs and/or enabling attractive business opportunities.
Digitalization of Business Processes
The business of companies is defined by their business processes. So it’s best to look at business processes first. These are usually the processes within operational functions:
- Marketing-process
- Sales Process
- Customer service process
- Product development process
- Production process
- Maintenance process
- Logistics process
- Financing process
- Other …
Once all functional processes have been conceptually captured, the next step is to identify the degree to which these processes are explicitly defined either verbally or in the form of flowcharts. In addition, objectives should be defined for each functional; if this is not yet the case, meaningful objectives should be developed, all of which should contribute something to the goal of the business process.
The decisive factor now is the extent to which the lived reality matches the documented process descriptions. Deviations indicate a lack of implementation discipline or poorly defined processes. The causes of such deviations should now be identified and eliminated.
However, even clearly defined and followed functional processes are no guarantee that the company as a whole will work efficiently and effectively. For this to happen, the functional processes must be meaningfully linked to one another. Only this results in a fully networked company-wide business process from which mutual dependencies emerge. Business process design is a complex process because the business process is usually complex. Simplifications in the business process representation that do not do justice to reality are of no use to the company. They lead to multiple work, bottlenecks and employee dissatisfaction. This is why a careful business process analysis and definition is so important. In particular, organizational interfaces must be carefully defined. Where operational processes cross departmental boundaries, expectations must be matched with opportunities and agreed upon. In order to stabilize the business process, it is particularly important to design feedback loops at the interfaces within and between processes, and also externally. This makes processes capable of regulation; they can adapt.
Finally, a performance review should be designed into the business process. In which sub-processes are the goals achieved, and in which are they not? Is there friction in or between the processes despite careful design? Corresponding adjustments of the functional sub-processes and thus of the entire business process should, by the way, be created as an ongoing task in the processes, because the environmental conditions are also constantly changing.
Only when the business process as a whole is in place and has proven itself in practice does it make sense to think about digitization steps. It is worth setting clear criteria for digitization:
- In which sub-processes could digitization directly save effort and costs?
- In which sub-processes could digitization relieve employees of routine work?
- In which sub-processes could digitization reduce the susceptibility to errors?
- Which costs due to deviations from the defined business processes could be avoided by digitizing the business process by executing the digital process steps directly from a digital workflow?
- Where could the consistency of the business process be improved by digitizing several sub-processes?
- Through which digitization steps could lead times be reduced?
- Which sub-processes could achieve a higher output through digitization?
- Which attractive business models could be enabled by digitizing subprocesses?
These guiding questions give an indication of the priorities for the digitization of business processes. Digitized workflows allow, for example, multi-stage approval procedures, but also the sensible coupling of processing tasks between manufacturing islands in production processes.
If you want to find out how well prepared your company is for digitization, you can use the “digital business process maturity model” developed by the industry association bitkom e. V. (https://www.bitkom.org/Bitkom/Publikationen/Reifegradmodell-Digitale-Geschaeftsprozesse).
Collection and Evaluation of Process Data
Each sub-process generates data on an ongoing basis. As a rule, this data is only partially used. If the data is already available digitally, it flows partially into companies’ ERP systems in order to create predefined evaluations and standard reports. However, if you specify correlations, you will also only receive results that are evident from these correlations.
There is much more information in process data that is often not obvious. Artificial Intelligence AI applications can identify surprising patterns in large amounts of data that help to really understand the business and take the right actions. Changes in patterns, such as a trend reversal, can also be detected by AI-based applications.
Such applications can be used, for example.
- for real-time monitoring of customer preferences and targeted offers,
- for situationally adapted, targeted customer care,
- for preventive maintenance to avoid machine downtime,
- for production planning coordination in plants with multi-stage manufacturing,
- for optimizing OEE in complex, multi-stage manufacturing plants,
- for on-time order completion and delivery
- and for optimization of inventory management.
These possibilities require digital acquisition of process data. For this purpose, the relevant machine components must be equipped with suitable sensors that record this process data and send it to an evaluation unit (CPU). This evaluation unit can make recommendations, but also make decisions independently within a predefined framework and implement them directly by issuing corresponding instructions to actuators. In this way, process data help to create a controlled, possibly even self-learning process.
IIoT
Applications for IIoT
Every device and every machine in the manufacturing process continuously generates data, but this data is often only used locally. By connecting the devices via the Internet (also called “Industry 4.0”), this data can be used throughout the entire system.
This adds intelligence to the monitoring and control of devices and machines. With the availability of many sensors collecting relevant data and actuators in the system, control processes not only become better, but can even be implemented and take effect in real time. Inefficiencies and errors can be detected at an early stage, and ideally even anticipated and immediately averted through the use of pattern recognition and artificial intelligence.
Typical areas of application for IIoT are:
- an even capacity utilization along the business process to avoid the disastrous “bullwhip effect”
- a reduction in delivery times
- a performance measurement and improvement in the individual stages of the value chain
- a streamlining of operations to increase overall efficiency in manufacturing processes
- effective process and product quality assurance
- a predictive ordering procedure for efficient inventory control
- preventive maintenance of components and facilities
- sustainable and environmentally friendly energy management
- traceability of process data throughout the entire production chain
- reliable and cost-effective asset tracking
- improving workplace safety through smart industrial glasses that use augmented reality to provide instructions on how to work
Components for IIoT
The Industrial Internet of Things (IIoT) is composed of components, devices, machines and plants equipped with sensors that can record operating data. This operating data can be shared with edge components via the Internet with other components and exchanged with computers that are integrated into the network. Actuators are also integrated that can be controlled by computers. The actuators can perform actions. In this way, complete manufacturing processes can be monitored, controlled and optimized using IIoT.
An IIoT infrastructure thus has sensors and actuators at its base. Both the sensors and the actuators are connected to the infrastructure in the network via edge components. The signals from the sensors are partially processed directly by the edge components or routed to IoT gateways or edge gateways; the actuators receive their instructions either directly from the edge components or from the IoT or edge gateways. The gateways are in turn connected via the Internet to the data server at the site (on-premise server) and to the overarching IoT platform. The available hybrid data is integrated and analyzed by business analytics applications. Edge technology ensures that many standard processes can be executed locally by the edge components. This speeds up and reduces the cost of processes. Less traffic has to be routed via the Internet to the data servers and vice versa.
Data is increasingly being exchanged using the 5G standard (up to 20 Gbit/s). This enables high data throughput in near real time. Since the range with 5G is less than with 3G/4G, it is advisable to install intelligent hubs in the network (edge technology). Then, with 5G, even spatially widely distributed components can communicate with each other in near real time. In combination with the analysis of big data, IIoT thus makes a significant contribution to the digitization of (industrial) processes and supply chains.
Vendors of IIoT Platforms
The following vendors of IIoT platforms are known:
- ABB Ability: IIoT applications with a focus on machine intelligence
- Cisco IoT Systems: platforms for network connectivity using edge computing
- Fanuc Field System: IIoT platform for linking disparate systems
- GE Predix: Platform for digital industrial applications
- Siemens MindSphere: Industrial IoT solution incorporating AI.
Companies should assess how high the security requirements for IIoT components and data transmission should be. With the selection of components and the data transmission standard, companies have an influence on the level of security. It should be noted that individual signals from components are hardly worth protecting, while the totality of operating and process data definitely contains information relevant to competition, is worth protecting and should therefore definitely be secured.
Cloud Computing
Storing large amounts of data in the company and maintaining and updating all possible applications in the company itself is time-consuming, expensive and involves risks with regard to availability and data security.
An alternative is the device-independent use of data, application and computing capacities efficiently and securely stored in server farms. In this way, even elaborate software applications such as AI tools, IoT environments, analytics applications and blockchain software can be used by companies without having to host them themselves.
However, a necessary prerequisite for this cloud computing is the software’s mandate capability via a multi-tenant architecture. This requirement was not met until the late 1990s. Providers counter fluctuations in workload with a service-oriented architecture.
Users access their own data and applications via a secure Internet connection using a mobile app or web browser. For users, it is irrelevant where the data and application servers are located. However, for data protection reasons, care should be taken to ensure that the servers are at least located on the user’s own continent. This alternative for storing corporate data and applications is called cloud computing. It is billed via a monthly flat rate or according to actual use – as Software as a Service (SaaS).
Cloud computing reduces the effort required for companies to maintain applications and data storage capacity and reduces the technical requirements for end devices as well as the service of user-side end devices. Secure access to applications can be provided via assigned user profiles.
Users can freely choose which resources they want to use when and with which end devices (on-demand service). Providers usually pool the use of their on-demand services in a distributed network of resources. However, users have no control over which provider resources are used to run their services. For users, capacities appear unlimited, while cloud computing providers can switch capacities between users as needed (rapid elasticity).
Segregation of Cloud Computing and Grid Computing
An alternative to cloud computing is grid computing. While in cloud computing the responsibility for the services lies with a specific provider, grid computing consists of the shared use of common resources without central control and responsibility.
Fog Computing
In fog computing, applications are chosen to be as close to users as possible to improve network efficiency and reduce latency for users. There is a conceptual similarity here with edge computing, which is used for the IoT.
Layers of Cloud Computing
Software-as-a-Service (SaaS)
If software applications are used in cloud computing mode, this is called software-as-a-service (SaaS).
Well-known examples of SaaS cloud computing are the Google Drive, Apple iCloud, Microsoft OneDrive and Amazon Web Services offerings. Microsoft’s usual workplace programs are also offered in cloud computing mode, such as MS Office 365. Finally, enterprise resource planning (ERP) systems such as Microsoft Dynamics and special production planning software, as well as other special business management applications such as Datev accounting systems, can also be used in cloud computing mode.
Function-as-a-Service (FaaS)
There is also the option for companies to book specific software functions. Providers then provide companies with exactly the functions they need. Billing is based on the functions booked.
This form of business model is also becoming established for modern cars: All functions, such as steering headlights, are built into the vehicle, but only the functions booked are activated for the vehicle owner. This creates standardization advantages for providers and individualization advantages for users.
Platform-as-a-Service (PaaS)
Even entire software infrastructure frameworks are offered web-based, for example for developer environments. Such platforms are called Platform-as-a-Service (PaaS). Examples include Amazon Web Services, Google Cloud and Microsoft Azure.
Infrastructure-as-a-Service (IaaS)
The top level of cloud computing is the provision or use of infrastructure, i.e. the services of a data center, firewall-secured access to a network and server CPU capacity as well as storage capacity (RAM, SSD). Such utility computing offerings are recommended for companies that do not want to operate their own data center.
Types of Cloud Computing
Private Cloud versus Public Cloud
Companies can choose whether they want to use an IT infrastructure as completely their own applications in cloud computing mode (private cloud) or whether they want to use a ready-made standard IT infrastructure via a public network with private access data (public cloud). In the first case, the company is responsible for setting up and maintaining the IT infrastructure; in the latter case, the company can rely on the provider to have a functioning infrastructure ready.
Virtual Private Cloud
A special form of public cloud is the “virtual private cloud”, in which a private area in a public cloud is sealed off from the public by a VLAN solution.
Community Cloud
A variation of the public cloud is the community cloud. A group of defined users receives access to services provided by a provider. The user group can be an industry cluster, for example.
Hybrid Cloud
In between, there are also mixed forms (hybrid cloud), with which competition-critical or specific core processes of companies are operated privately and other generic processes are utilized via flexibly scalable public clouds.
Hybrid cloud offerings for enterprise applications are likely to become established.
Multi Cloud
In a multi-cloud solution, access to various cloud offerings from different providers is bundled in such a way that users are unaware of it. All offerings can be used with the same access data.
Data Integration
In the future, companies will process both structured and unstructured data in different data formats and from different data sources from different companies to obtain information that is relevant for the business. They must be able to simultaneously read, integrate, synchronize, process, evaluate and move such “hybrid data” in real time from clouds, their own and third-party data stores. For this, companies need suitable architectural patterns, methods and tools. Data integration tools that have these capabilities are commercially available.
Requirements to Data Integration
The functional requirements for commercially available data integration applications are as follows:
- Ability to move data physically and virtually, uni- or bidirectionally, in (micro-)batches or in real-time.
- Ability to direct queries to different (virtual) data sources using adaptors
- Ability to move data, either in discrete units (events) or as a running stream (data streaming)
- Ability to handle data movement via inbound and outbound APIs as “data-as-a-service”.
- Ability to perform complex data analysis using text or data mining or modeling.
- Ability for data sets to “self-heal” through the use of metadata and learning capabilities
- Possibility of preparing data sets for analysis by users from specialist departments without programming knowledge
- Possibility of secure data transfer within a hybrid data infrastructure through “containerization”.
For medium-sized companies, it is advisable to book the data integration service with specialists instead of configuring and customizing data integration applications themselves and using them for their own use cases.
Vendors of Data Integration Services
Experienced providers include
- Amazon Web Services (with AWS Glue): Logistics companies, e-commerce companies and others
- Denodo: financial services, manufacturing and technology companies
- Hitachi Vantara: Financial services, software and technology companies, consumer goods manufacturers and retail: Hitachi Vantara also offers a platform for Industrial IoT with Lumada Industrial DataOps.
- IBM: financial services, insurance, healthcare companies,
- Informatica: Financial services, telecommunications, public sector.
- Microsoft: Small, medium and large enterprises across all industries.
- Oracle: financial services companies, telecommunications companies, e-commerce companies, pharmaceutical companies
- Precisely: financial services, insurance, and healthcare companies
- Qlik: financial services, healthcare companies, retail and manufacturing companies
- SAP: automotive industry, consumer goods manufacturers, public sector
- Software AG: financial and insurance service providers, technology companies, telecommunications companies.
- Tibco Software: financial services providers, telecommunications companies, manufacturing companies.
These vendors use frameworks such as SQL Server Integration Services (SSIS) for on-premises databases and Azure Data Factory (ADF) for hybrid data sources. Oracle has been involved with database solutions for a very long time, using its own Oracle Golden Gate platform, Oracle Data Integration Suite (ODI), Oracle Big Data SQL (BDSQL), and integration services within Oracle Integration Cloud and Oracle Cloud Infrastructure (OCI)
Deployment areas show that most of the data integration effort is in financial and insurance services, telecommunications, healthcare, retail and the public sector. Some vendors also have solutions for logistics and manufacturing companies.
- A suitable provider can be found for every industry and every company size.
- It is also important not to limit data integration to your own company, but to create opportunities to extend data integration to the entire value chain.
- When selecting, make sure the vendor understands your business and can and wants to cover your requirements.
- Look carefully at the vendor’s business model to see if it fits your needs.
For most businesses, it is not advisable to implement open source frameworks yourself or use programmable interfaces.
As a user, you should rather focus on the analyses of the data.
Big Data and Business Analytics in industrial Processes
The amount of data available is growing exponentially. This seems like a curse to many companies because the volumes of data have to be managed. But a lot of available data can also be a blessing for companies, because it contains relevant information that can be used advantageously for the business. Unfortunately, it does not remain an option, because many companies already know how to evaluate and use Big Data very well. For the other companies, this triggers the compulsion to now also deal intensively with Big Data.
Development and Significance of Big Data for Industrial Processes
Since the availability of personal computers and client-server computing, process data from various data sources has been accumulating in large quantities, in various formats and at high speed. These three criteria – volume, variety and velocity – characterize Big Data. Data sets can become correspondingly complex. Such data sets contain a lot of relevant information that can contribute to better decisions. But unfortunately, this information is not obvious. Even relational databases, combined with Structured Query Language (SQL), are not capable of meaningfully evaluating such data volumes in real time. The Internet with its search engines and Internet-based transactions (digital economy) have contributed to a further increase in the amount of data generated.
Proprietary data warehouses have emerged in companies to manage, analyze and utilize these large data volumes. With the advent of social media, however, the volumes of structured and unstructured data generated by users then multiplied. Storing the huge amounts of data at a reasonable cost and simultaneously processing this data distributed across many computer clusters became particular challenges for companies. It was not until the data processing speed of computers evolved, arbitrarily scalable storage became affordable with virtual clouds, and reliable data streaming became possible that artificial intelligence (AI) applications became prevalent and learning machines (ML) emerged. While data had to be extracted, cleansed, transformed and aggregated (ETL) before it could be analyzed, raw data can now be stored and fed directly to the analysis. It is only through this that data evaluations, data-based decisions and the initiation of data-based measures become possible in real time.
Infrastructure and Data Management Tasks
The tasks can be divided into infrastructure tasks and data management.
Infrastructure Tasks
The infrastructure tasks consist of storing the data in its original formats, integrating all public and private data sources, providing tools to access the data (search, explore, govern) and enable data steaming, and providing evaluation applications (query tools) and applications for data analysis (business analytics) that can be used to identify trends and work out making decisions.
There is a great opportunity for companies to have the infrastructure task of data warehousing performed by specialists in the cloud and to focus on business-related data management.
Data Management Tasks
It is important for every company to evaluate structured data from the data warehouse as well as structured transaction data. In order to understand changes in markets and customer preferences and to develop suitable products quickly and in a targeted manner, there is an increasing desire to also evaluate unstructured data from social media and other sources that are available in so-called data lakes. The information hidden in Big Data can be tapped through Business Intelligence (BI) applications. The term “business intelligence” was coined by IBM in 1958 and refers to the ability to identify interdependent relationships between facts and use that information to take appropriate action to achieve desired goals. It is essentially about combining data and recognizing patterns in data sets. In order to gain valid insights, data from all three sources, the data warehouse, the transactional data pool and the data lakes, should be analyzed in an integrated manner. Data Platforms are available today for this data integration.
Artificial Intelligence (AI) applications can now be used to perform much more extensive analysis: AI can describe images, analyze data graphically, transcribe spoken text, read aloud written text, and extract meanings from statements broadcast on social media. Embedding such artificial intelligence applications in Data Platforms enables companies to understand stored data in a business context, unlock tacit knowledge for the business, and create entirely new applications. These can be applications for process optimization, inventory optimization, capacity utilization optimization, quality monitoring, preventive maintenance of assets, or identification of particularly loyal customers or those at risk of churn.
Data management is an important strategic task that companies should not abandon, because this is their opportunity to position themselves advantageously in the competitive environment. The challenge is to find and retain qualified employees who are familiar with the methodology of data management and continuously update their skills. However, it also makes sense to involve experienced service providers in the task of data management. At least in the conception phase, this makes sense in order to implement a suitable methodology. In addition, it can be helpful to use an external data management specialist as a sparring partner.
Robotics
The field of Robotics encompasses the design, development, construction, programming, operation and maintenance of robots. Robots are changing the skills that humans should have. While simple jobs are increasingly performed by robots as wage levels rise, employees must be able to build, program, operate and maintain robots. The range of tasks and with it the requirements profile for employees is shifting toward computer science, electrical engineering and mechatronics.
Machines can help employees carry out defined machining operations, while automatons can even be programmed to carry out operations independently. Robots, by definition, go one step further: they are mobile and work to fulfill goals; to do this, they can orient themselves in their environment and even decide and execute various operations independently.
For a long time now, not all work in production and logistics environments has been performed by humans. For repetitive routine tasks, for work with high precision and/or speed requirements, and for dangerous work, machines, automats, or even robots are used, provided they are profitable. There are certainly tasks that are reserved for humans simply because robots are too expensive for them. The use of robotics therefore always requires a use case and an investment calculation.
Criteria for automation include the quantities to be processed as well as the degree of standardization of the work. In the case of series to large-scale production, automation by robots is more likely to be worthwhile than in the case of single-item production or small machining batches. There is often a requirement to produce large quantities with a large number of variants. This often results in very small production batches. But even such order structures can certainly benefit from targeted automation if the components are standardized and the products are modular. The concept of automation therefore requires the course to be set in a suitable manner much earlier in the product creation process. The later the variants can take effect in the manufacturing process, the more modularized and automated manufacturing can be. Of course, such a change process is not purely a manufacturing issue; rather, product management, product development, procurement, work preparation and production planning and control are all involved. After-sales service can also benefit from a newly planned product design in that less variety of spare parts has to be kept in stock and wear parts may be easier to replace.
Production Line Automation
When it comes to project ideas for automating processes, the question often arises as to what should be done first: digitization or automating the processes? This is not a trivial question. After all, both activities are mutually dependent. That’s why both tasks should be tackled in parallel.
However, uncompromising automation is no more a panacea than uncompromising digitization. In the automation of entire production plants or production lines as well as in automation in the process industry, it is necessary to optimize a trade-off between maximum efficiency and a necessary flexibility. Machines fully networked to form a production line can achieve high efficiency – as long as everything really works. Small disturbances can completely destroy line efficiency. Therefore, it may be expedient to keep buffer stores between the processing machines.
The better the process data of such a production line is known, the more likely it is that malfunctions can be counteracted in advance in order to limit downtimes.
- In any case, it is worthwhile to accurately record the type of business process, because the selection of machines and equipment depends heavily on the natural business process. An attempt to comprehensively automate established workshop processes is very likely to fail.
- However, with growing workshop operations, it may make sense to initiate a shift to industrial processes. If this seems feasible, it is also worth considering automation.
- The performance of the components should be coordinated. It should be noted that the weakest link in the coupled system determines the efficiency of the entire line.
- As a “law of nature”, line efficiency decreases with the number of linked components. In this respect, the complexity of the line must be thoroughly scrutinized already in the planning phase.
- The performance of a linked plant depends to a large extent on the data communication between the components. Therefore, the analysis of process-relevant data, the implementation of data acquisition via sensors as well as data transmission and evaluation in the network are important.
Appropriate considerations and analyses can provide valuable and robust insights that can be used to make a decision on partial or step-by-step automation.
For the automation of plants, the machine controls must also be integrated. In some cases, the migration of control systems of existing plants to the new automation technology is an option. A thorough knowledge of the relevant machine controls is therefore indispensable. Common machine controls include:
- ABB 88xA, Freelance 2000
- Emerson Delta V
- HIMA
- Honeywell PKS, TDC 3000
- Mitsubishi
- Siemens S5, S7, PCS7, PCSneo, TIA
- Yokogawa Centrum VP
It is recommended to involve machine control specialists in automation projects.
Computer Vision
“Computer vision” is another interesting field for making manufacturing processes safer and more efficient and cost-effective. The term “computer vision” refers to the ability of computers to understand image data and make meaningful decisions.
Similar to humans, computers can be trained to reliably distinguish between different types of objects, perceive movements, estimate distances and identify deviations from a target state. Cameras, data transmission and evaluation algorithms take over the tasks of the retina, the optic nerve and the visual cortex. Computers have to be trained for differentiated perception in the same way as humans. Particularly important is the definition of criteria with which, for example, good goods can be distinguished from rejects. Humans also need to be conditioned to this. Machines need hard criteria that can be recorded in the form of algorithms. With “experience”, machines also learn to distinguish better and better (deep learning). This independent learning can even be extended to include a predictive capability through the use of a Convolutional Neural Network (CCN). Both work not only for static images, but also for video data.
Thus, computers can not only replace employees in many use cases; they can even process inspection and separation operations much faster with consistently high concentration than humans can.
This is why computer vision applications in manufacturing processes are suitable for ongoing quality assurance. Together with the monitoring of machine parameters, computer can be used to avoid failures and rejects.
Of course, computer vision applications do not come for free. To be sure for which processes an investment in this technology is worthwhile, both a process analysis and an investment calculation are recommended.
Digital Twin
Everything that exists in the real world can be modeled in digital form. This means that manufacturing processes can also be mimicked digitally. Realistically functioning models of processes can be used to simulate process changes and assess the effects of these changes on the behavior of the simulated system.
As a rule, simulation on such digital twins is more cost-effective than trials on real systems. Manufacturing runs do not have to be interrupted for testing, and no physical input material or process energy is consumed.
Creating digital twins can therefore make sense, but it also involves a certain amount of effort.
However, digital twins only become really interesting when process data is exchanged between the real plants and their digital twins. Digital twins can then be used as a management unit that prescribes process adjustments to the real plant in order to be operated close to an ideal state.
A prerequisite for such communicating digital twins is the implementation of the IIoT concept. The process-relevant data must be made available by the real plant to the digital twin for matching. The digital twin must have the ability to control the actuators of the real plant.
Digital twins can also be helpful for other operational functions, such as agile manufacturing control, preventive maintenance. Outside of manufacturing, digital twins can also be used for intelligent and efficient control of warehouses and other goods handling areas (e.g., port logistics).
However, this concept of digital twins is not limited to manufacturing processes; it can also be applied to products, which can be simulated throughout their lifecycle as 3D geometric models, functional models or behavioral models. In this way, valuable insights can be gained without having to test physical prototypes. In particular, the consideration of the entire life cycle in the simulation (from the extraction of raw materials to the manufacture and use of the product and its possible secondary use to the disposal and separation and reprocessing of the raw materials (recycling) is gaining special importance in the wake of the compulsion for sustainable management.
The supreme discipline is achieved when different models are linked together in a simulation. Then the complex reality can be best recreated.
Digital twins are powerful tools to further develop real processes and products. An on-site analysis can show whether it is worth taking the first steps with digital twins.
Summary
With the use of digital technologies, relevant effectiveness and efficiency potentials can be tapped in industrial environments, especially in manufacturing and logistics.
A necessary prerequisite for this is a strategically sound digitization of the essential business processes and a digital recording and networking of the influential process data (IIoT, cloud computing). For both, a careful on-site analysis is recommended, which should already include what the data is to be used for.
The next step is to deal with the large amount of partly structured, partly unstructured data that is constantly being generated (Big Data) in order to gain valuable insights from the data (Business Analytics). Important steps for data evaluation are the way in which data is networked and data integration.
Now, beyond machine support in the execution of individual industrial operations, automation of entire manufacturing processes can be initiated. Agile control is now possible through data-integrated process control, leading to higher throughput, higher reliability and higher cost efficiency.
In addition, AI-assisted self-learning computer vision applications can be used in industrial processes for quality monitoring. This can improve the quality level and reduce the costs of quality assurance.
Finally, the concept of digital twins allows processes and products to be modeled realistically and adaptations to be simulated cost-effectively. By exchanging data with real processes, digital twins can be used to control real processes. With each use, the application learns and further improves the control process.
For classic industrial and logistics companies, it is financially worthwhile to look into digital technologies and make better use of available process data.